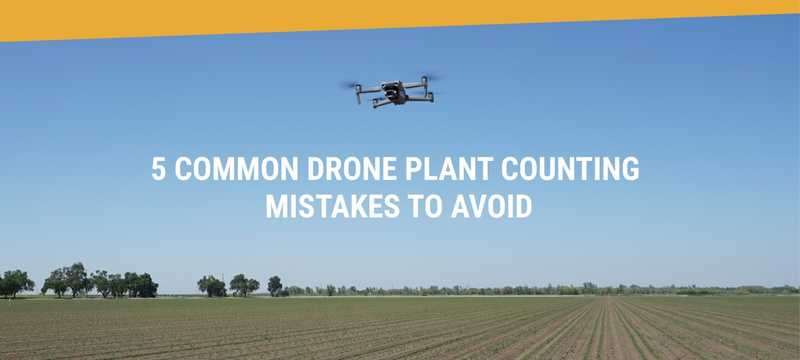
5 Common Drone Plant Counting Mistakes to Avoid
May 16, 2023
Drone plant counts provide a full field inventory that can drive precision management decisions. But your decisions are only as reliable as your data. Garbage in ⇒ garbage out. Drone imagery can provide fast and accurate crop stand counts if you know which pitfalls to avoid…
Because Solvi’s Plant Counts tool provides a visible preview (for free!), you can quickly gauge the accuracy of plant detection. If the proposed results aren’t quite right, you can adjust the model training to improve accuracy before initiating plant counts.
At Solvi we have processed plant counts in thousands of fields and have noticed the same patterns that compromise the accuracy over and over again. Here are five common mistakes that can tank your plant counting accuracy (and how to correct them!). We think you’ll notice a pattern.
1. Poor image resolution
Plant counting tool uses AI-algorithms that depend on the detail and clarity of your drone imagery for accurate counting. These algorithms are high-speed pattern matchers and prediction makers. So image resolution - or the quality of the image - plays a big role in how accurate your plant count will be. Remember that image resolution is a function of your camera specs and flight altitude. (Review our post on How High Should I Fly? for a primer on resolution.)
How High Does Image Resolution Need to Be?
It depends on what you’re counting. Large, well-defined plants, like trees, are distinguishable even when an image is slightly pixelated.
Even at 120m/400ft, the tree canopies are clearly visible for you to select and the algorithm to match.
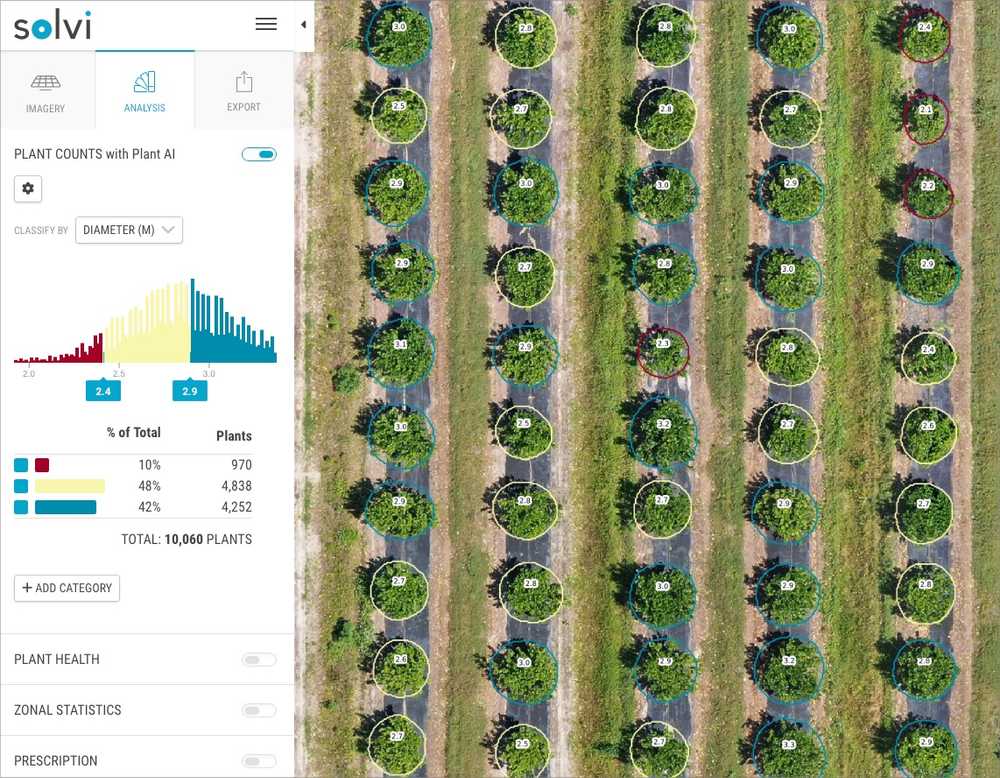
But smaller and less distinguishable crops require greater image detail to differentiate. Pixelated images (from high-altitude drone flights or some satellites) obscure detail making it tough to tell individual plants apart or from other landscape features, especially if plants are small, crowded, or overlapping. This can result in a significant over or undercount, which could cause problems down the line.
High-resolution images give a detailed look at your field and crop, including inorganic features, leaf shape, and leaf color, which improves counting accuracy.
We find that smaller plants are most accurately counted below 0.50 cm/px or 0.2 in/px. There are diminishing returns with higher resolution though, more detail isn’t always better.
High-resolution images take longer to gather and process and take up more space. So finding the right balance between resolution and practicality is key to a manageable workflow.
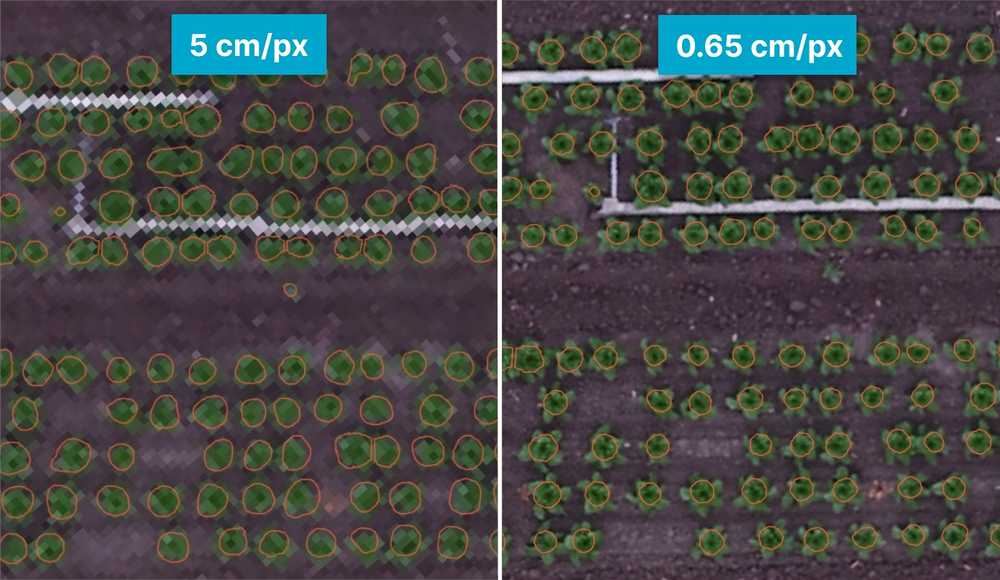
How to Fix Image Resolution:
- Take a few sample images at different altitudes before the flight and evaluate the clarity of each plant canopy in these images. Are the plant edges and unique plant-pattern well-defined enough for you to precisely circle sample plants? If not, try lowering your drone flight altitude. But keep it reasonable for your plant species and growth stage. For efficiency, we recommend flying at the highest altitude to accomplish your mission goals.
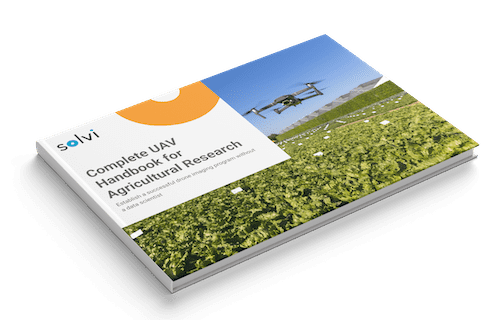
Download The Complete UAV Handbook for Agricultural Research
Learn how to establish a successful drone imaging program without a data scientist
Get your free pdf2. Wrong Growth Stage
Closely linked to image resolution is a crop’s growth stage. Plants at different growth stages may exhibit varying leaf sizes, canopy densities, and overall visibility. Visible defining details are necessary for the most accurate counts.
Small-Leaf Crops and/or Early Growth Stages
Small-leafed crops like corn or soybeans are tiny in the early stages. Pixelated images at this stage can confuse plants with weeds, mask inorganic objects like rocks, and obscure time-of-day issues like shadows.
But often, timely plant counts are critical for economic decisions like replanting. So, how do you ensure the most accurate counts with tiny plants?
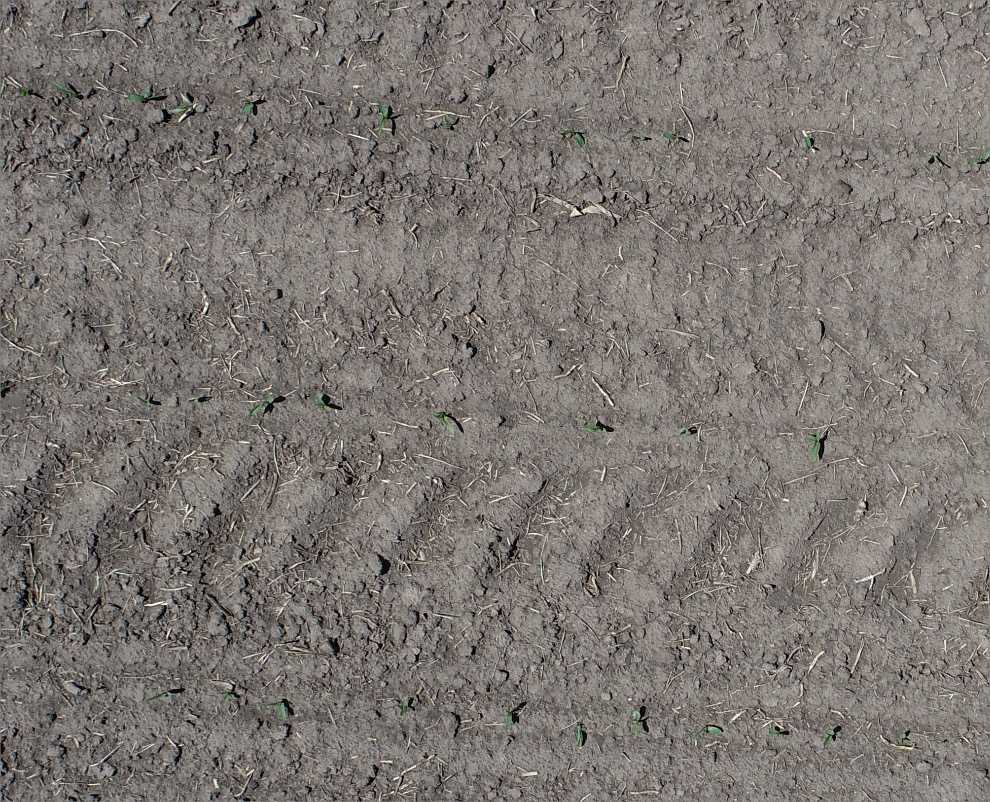
How to Fix Early Growth Stage Issues:
- Choose right timing for the flight. Whenever possible, choose a slightly later date for the data collection so that the plants get bigger and form a shape that easier to identify in the imagery.
- Review image resolution. If flying later is not an option, try to compensate it with higher resolution. Aim for 0.50cm/px or higher. For many drones, that will be an altitude of around 20m. But some newer sensors can shoot higher-resolution images at higher altitudes. Check your camera specs.
- Expand your sample area. Try enlarging your sample area to include more off-target items: tire tracks, cloud shadows, rocks, weeds, or skip areas. Include these in the sample area but do not select them as plant samples, and you’ll train the algorithm to ignore them.
- Select more plant samples. Within your field sample area, circle more on-target plants. This is especially important if plants have similar but different leaf shapes or colors. Show variation in sample plant sizes, shapes, and colors. More acceptable selections help broaden the algorithm’s selection parameters.
Dense Plant Canopies and Late Growth Stages
Larger plants often overlap and mask all boundaries making them difficult to count. For most agronomic purposes, early-stage counting is most practical and impactful on dense cereal crops for decisions on replanting, weed thresholds, and fertility management.
But late-stage counting can be useful for harvest timing and predictions in many specialty crops like cabbage or broccoli. Since these large plant canopies are often co-mingling with neighbor plants, image resolution is again your best insurance for accurate counts and sizing.
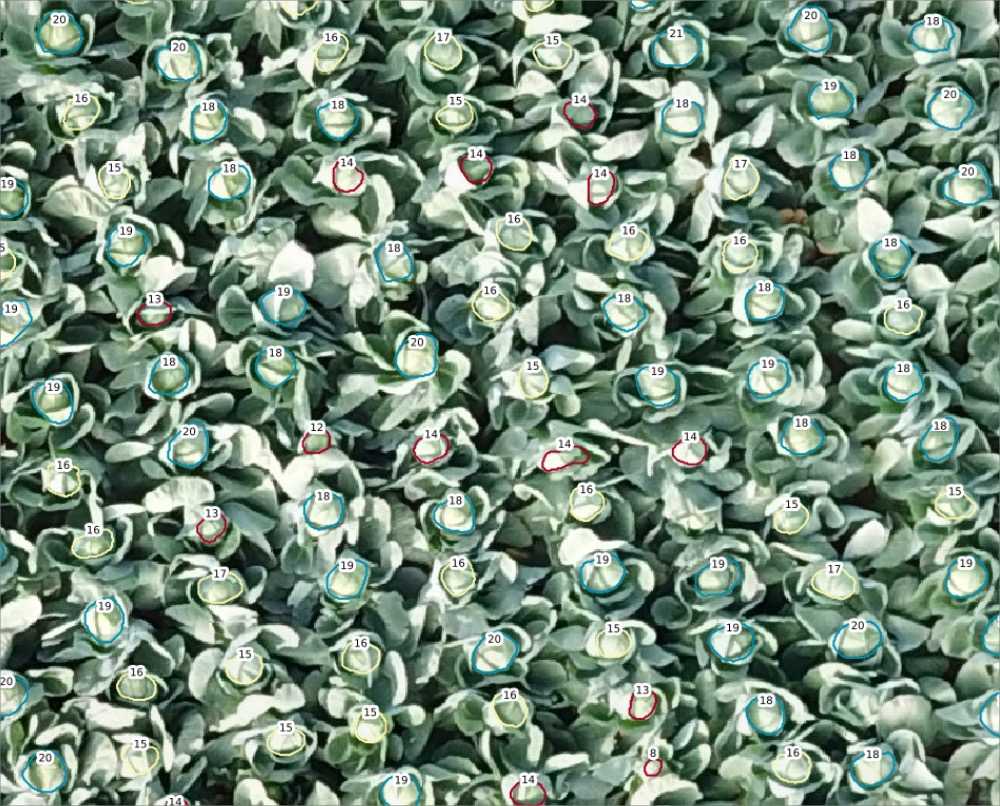
How to Fix Late Growth Stage Issues:
For crops where late-stage counting is relevant, verify your image resolution for the highest possible level of detail. Since specialty crops are often grown on smaller acreage than grain crops, flight times from low altitudes are less of an issue.
Also, consider the precision of your sample circles. Interested in head size? Limit your sample plant circles to the very edges of the head, not including surrounding leaves.
3. Not Enough Training Data
Solvi’s automated plant counts depend on a well-trained computer algorithm. Generally, providing more data with more variation renders more accurate count results.
PlantAI tool recommends a minimum of 10 example plants in your image sample area (blue rectangle). Choosing the minimum number of plants in a single row may yield reasonable results in images with a clear, weed-free background. But expanding your example set takes only seconds and can dramatically improve count accuracy and reduce the number of false positives (mistaken plant detections).
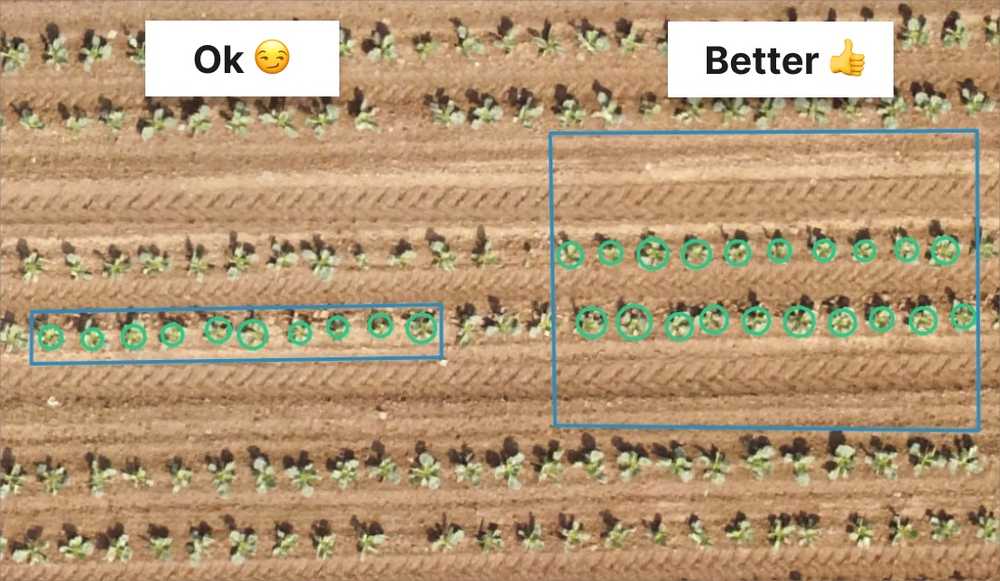
How to Fix Not Enough Training Data
- Expand your image sample area (blue rectangle) to include some area between rows and plants, especially bare, weedy, or rocky areas.
- Add 10-20 more plant examples. Include as many outliers in size, scale, or color as needed. Plant examples can be scattered across the sample area, not just from the same row.
- Iterate. Start with one example set and preview any inaccuracies. If necessary, add another example set to refine the count. Evaluate again and repeat until you are satisfied with the results.
4. Unwanted Detections
Fields are full of non-crop things! While it’s obvious to us that a tramline or footprint isn’t a plant, remember that the algorithms are performing high-volume pattern prediction and matching. Even if it’s a moon rock, if it looks like the target plant shape, it could be counted.
At a more sophisticated level, weeds or mixed crop varieties can be difficult to differentiate. While you likely want the weeds to be excluded from counts, if you have a mixed field of lettuce varieties, you may want all of them counted.
If your plant counts include unwanted items, like weeds, rocks, shadows, or if the counts are missing crop variations, the algorithm needs more training.
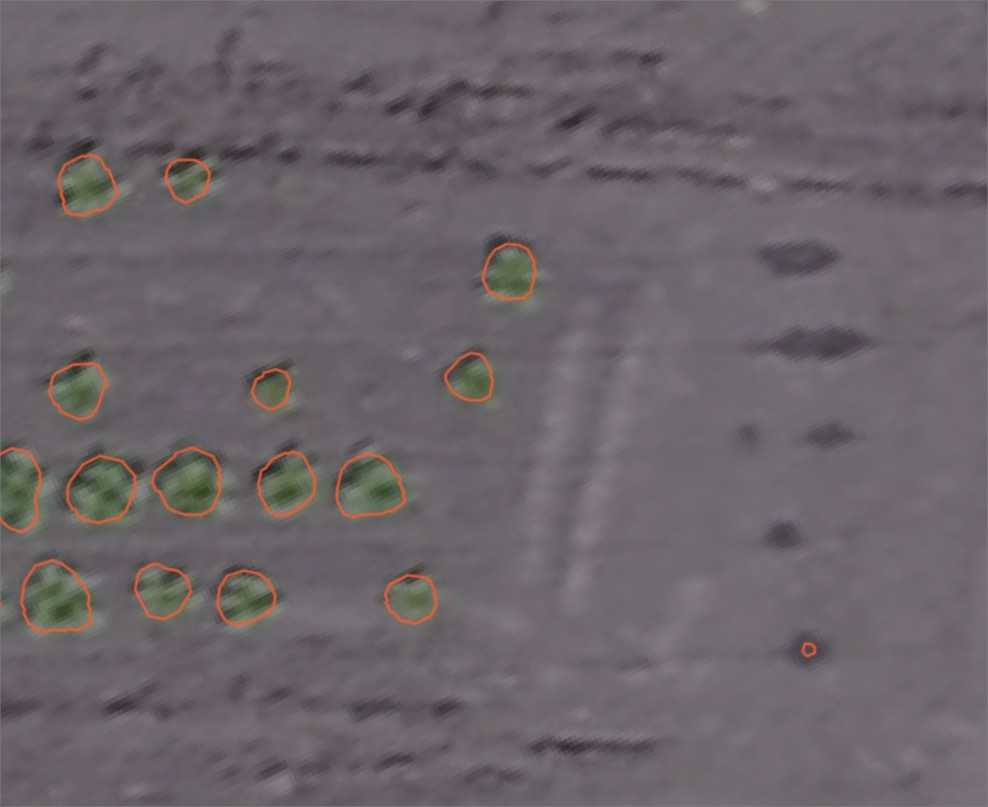
How to Fix Unwanted Detections:
- Check your image resolution. If imagery isn’t fine enough to detect leaf shape, it can literally blur plant/inorganic detection. Revisit the section on image resolution to ensure your camera specs match your crop and imagery needs.
- Change the sample area. It’s important that your sample box include any unwanted features. By including objects like rocks or weeds but not selecting them as samples, the algorithm will learn to ignore them. Relocate or resize your sample area box as needed to include a few examples of off-target features.
- Select a greater range of sample plants. If weeds are being counted, try selecting more target plants with size, leaf shape, or color variation. This provides more clarity on acceptable target plant parameters.
5. Missed Plants
If your plant counts are missing on-target plants, consider the amount of crop variation in the field. Real-world plants are not exact replicas. Because you train Solvi’s plant counting algorithm, it’s as sensitive as you train it to be.
Within your image sample area, we recommend selecting at least 10 target-plant examples. But if there is a significant range of plant sizes, shapes, placement, density, or colors, the algorithm needs expanded training.
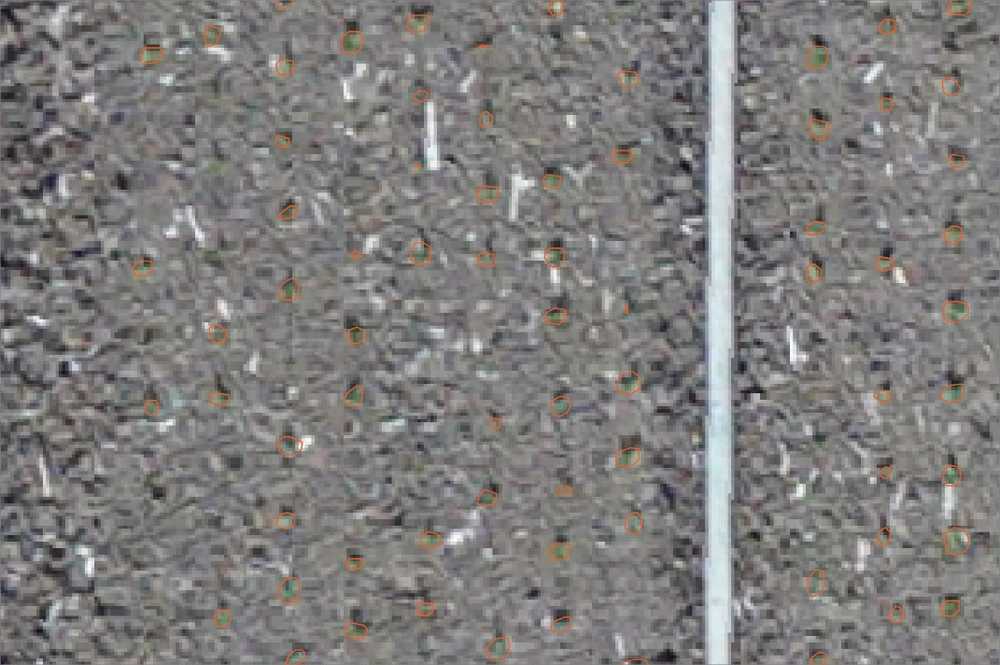
How to Fix Missed Plants:
- Check image resolution. Ensure that resolution is sufficient to distinguish plants in their growth stage.
- Select more example plants. While 10 examples is our recommended minimum, adding more example plants will further refine the algorithm’s selection criteria. But keep it manageable. The intent is to save time!
- Choose a wider range of plants. Make an effort to include on-target plants that represent small/large, dense/loose, damaged/healthy, regular/irregular shapes, or multiple colors depending on your interests. Increasing the acceptable parameter range can refine the algorithm’s counting model for more accurate results.
The purpose of AI counting is to save time and improve accuracy. But a few minutes of well-planned algorithm training will reap great rewards in output accuracy.
Agricultural Field Expertise
At Solvi, we’ve counted thousands of crop fields for growers, agronomists, and agricultural researchers around the world. Our sophisticated plant counting algorithms were built by and for agricultural research, making them the most accurate available.
Leading breeding and research organizations, including Rijk Zwaan, Agrovista, Nordic Beet Research, the Swedish University of Agricultural Sciences, and others, use Solvi’s image-stitching and analytics tools to improve their data collection speed and accuracy.
Looking to extract plant-level data from your drone imagery? Try counting your entire field with Solvi for free today.